Our client had a slow and laborious process for making data inquiries that often took a week or more to turnaround. Follow up questions on these inquiries took just as long, creating a frustrating and inefficient process, which was unacceptable for such a premier enterprise organization. They had tried cloud-based solutions previously and met with little success. At the speed business flies today, they needed to find a way for management to become self-service business intelligence users.
The challenge
Because data was spread across the organization and lacked a central source of truth, data inquiries required a request be placed with the data engineering team. The team would build a custom dashboard to display the data in a useful manner. The turn around from inquiry to analysis was about a week and required significant work hours to complete.
Not only was this process arduous and time consuming, it often suffered from a disconnect between the high-level business goals of stakeholders, and the hyper-focused requests made to engineering.
The solution
We started by interviewing multiple stakeholders in order to understand the business needs of the organization and the capabilities of their data engineering team. We quickly learned that disparate data was siloed and different departments within the organization used a piecemeal collection of non-compatible technology tools and methods to collect, store, quantify, describe, and analyze their data. They lacked a cohesive and holistic overview. This is something we’ve seen time and again in some very sophisticated organizations.
The solution was to create a complete end-to-end next generation data analytics platform. While this went well beyond the originally stated problem, the solution gives them a complete view of their data and each stakeholder gained the ability to independently perform their own analysis and reach meaningful, actionable insights.
Data & Cloud Analytics Strategy
We needed to fix their cloud data warehousing—in other words, get them out of the siloes and into the pool. We started by recommending Snowflake for their data warehousing needs. Snowflake allowed us to achieve the kind of flexibility and speed needed to solve their business goals most efficiently.
Snowflake provided them with a scalable platform that allowed us to migrate data from spreadsheets, other cloud storage solutions, and a variety of legacy products throughout the organization into a single, consistent place.
Data Analytics Applications
Setting up a data pipeline and transformation flow was critical. We set them up with Airflow, an industry standard data pipeline and then built out additional pipelines for data transformation. The pipelines gather data from across the organization, centralize it, and bring it into Snowflake.
This gave them a holistic view of all the different steps needed to collect and centralize their data and made sure that each component is running in the correct order with the correct dependencies. We used Airflow due to its centralized alerting, monitoring and logging, and flexibility.
Self-Service Business Intelligence
Better questions lead to deeper insights. We put Looker on top of Snowflake to empower the research teams to gain holistic access to all of the data they need to answer their toughest business questions. The spreadsheet paradigm was rendered completely obsolete.
Most importantly, the research teams were able to use the Looker dashboards we built for them to answer a variety of questions without the need to involve the data engineering team. This not only allowed the research team to serve up their own inquiries, it had the additional benefit of freeing up IT to perform other tasks more suited to their capabilities.
Now that the research team had access to the information more holistically and in a centralized place, they could quickly ask and get answers to follow-up and more sophisticated questions.
In training the business team to become self-sufficient, we had to adjust to the company’s business needs as the project was happening. Initially, we were looking at 25 datasets. Once the business teams understood their ability to get a holistic view of the company, we were able to focus on the five largest and most valuable sets, giving the company the most bang for their bucks.
Data Governance
Data governance provides a unifying truth, and one aspect of working with Looker is data governance. It’s impossible to analyze data unless data definitions, metric definitions, and dimension definitions are centralized. Looker is built to emphasize consistency.
A unique piece of this project was show names. Different departments named the same show differently. Let’s call one of their shows; The Primetime Show. It could be listed in their database in a number of ways including: “The_Primetime Show,” “Primetime Show, The,” “ThePrimetimeShow,” etc.
Just the naming conventions alone made it a challenge to pull the data they were looking for. So if you want to know something as simple as how many people watched the show last week, you could get three widely different results depending on which data set you were looking at.
To solve this problem, we built out a process that uses natural language processing—a kind of advanced analytics machine learning technique—to automatically match show names together, consolidate them, and standardize them so that a user can see all three rows even if the data entry isn’t consistent.
Data Platform Modernization & Migration
Finally we needed to bring it all together. A modern, well conceived data analytics platform allowed us to consolidate data from a variety of legacy tools and piecemeal cloud storage options into a single database. We were able to migrate an incredible amount of disconnected data to the cloud and create a database that is now a useful resource for the people who need it the most–the research teams and managers who rely on the data to keep their edge in the competitive media industry.
How did it work out?
The time to insight has improved from one week to one day. All of the steps we took and the technology we employed have transformed the research teams. Once frustrated slow-moving analysts, dependent on data engineering teams to interpret their needs, they are now self-service business intelligence professionals able to look deeper into their data, ask more challenging questions, and get results at a speed that makes sense in the current competitive business environment.
Services provided
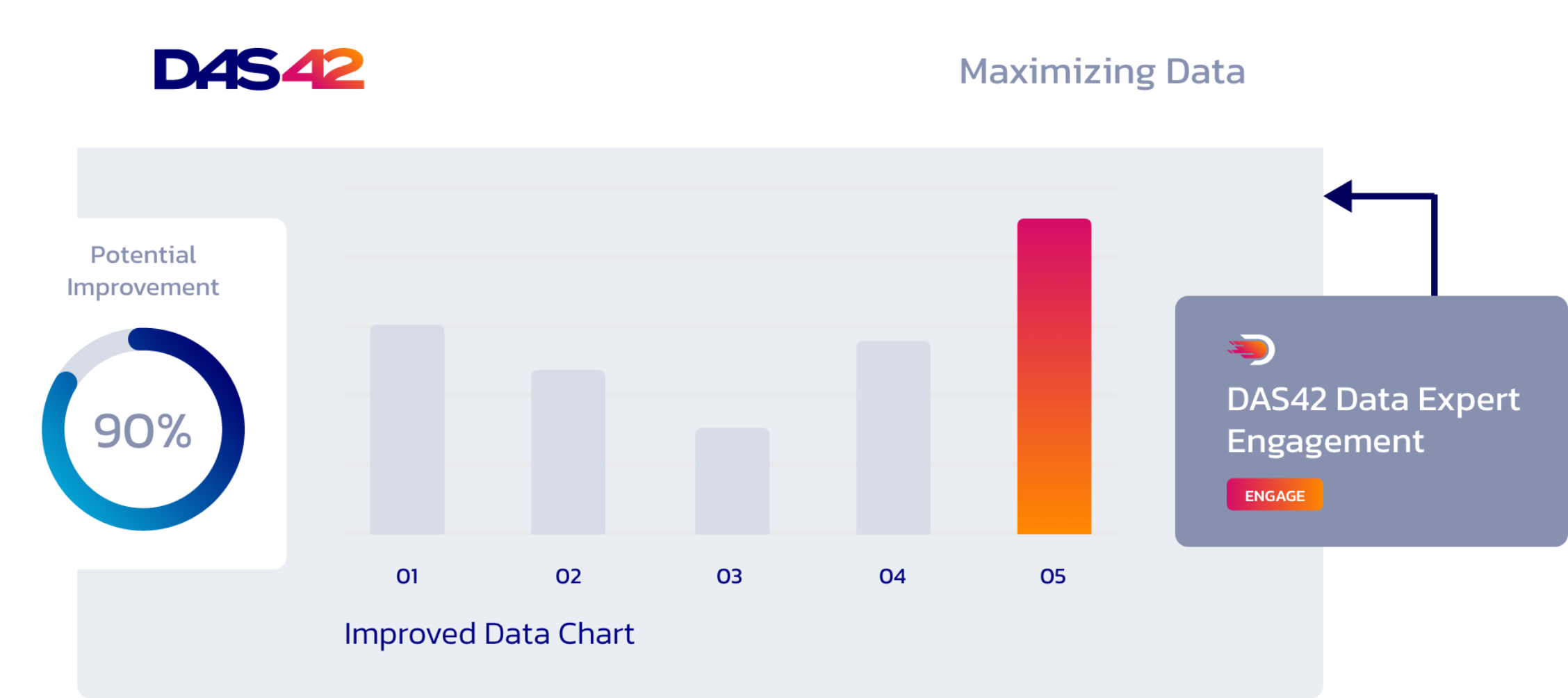
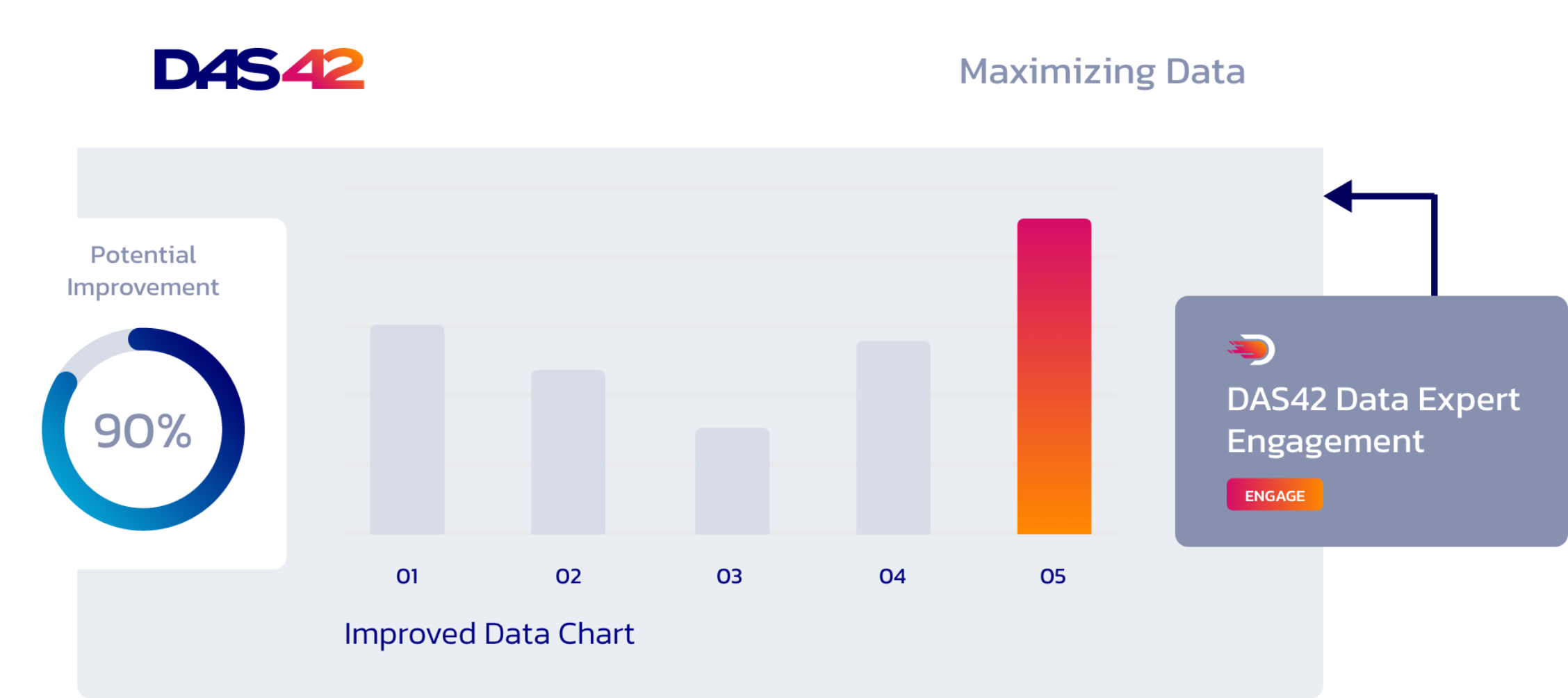