How to structure your data teams throughout your organization’s analytics journey
No matter the size of your organization, implementing a modern data analytics program is no small feat. Along with managing the nuanced technical demands of a data stack, you need to have the right structure in place to allow critical areas of your business to function efficiently.
And, unfortunately, the organizational adjustments don’t stop there.
You need to integrate both your data and its support system to ensure your data modernization initiative is sustainable for the long term. Will your organization be best served by retaining a centralized data team that serves every business unit, as you may have done with your previous processes? On the other hand, maybe embedding an analytics expert with each team will better serve your needs. Or maybe your goals are best served by a solution that lies somewhere in between.
The right answer ultimately depends upon where your organization stands in its data journey. And, just as importantly, where it wants to go.
A centralized data team gets your organization started right
If your company is relatively small and just starting its data journey, you may only have a single analyst to serve every team. Capable of serving multiple stakeholders, this “jack of all trades” provides data engineering support as well as insights into your organization’s performance across critical areas.
Typically, marketing and sales teams are among the first to be served by rolling out a data analytics program. But as time goes on, you need to extend your return on investment into new areas of your business. Your finance team may be looking to close the books faster, or HR may want to modernize to improve recruitment efforts.
Very quickly, your organization needs multiple generalists to effectively serve your data needs.
Simplicity is among the advantages of a centralized data team
Finding analysts that can mix the business and technical aspects of your data stack isn’t always easy. But with the right team in place, your centralized data team provides a vital resource that’s both cost-effective and straightforward to manage.
A centralized data team fields and prioritizes project requests from across your organization. From a management perspective, your data team’s leadership can clearly understand what everyone is working on and identify any duplication of effort. When analysts develop best practices for your sales team, their insights are readily shared and applied to processes elsewhere in your organization.
As your centralized team develops standards around data reporting, they’re easily communicated and deployed where they’re needed. However, as your company grows, the demands placed on your centralized team will increase as well.
Bottlenecks and competing concerns slow centralized data teams
As your organization grows into your data program, a centralized team can be bogged down with demands. Retrieving data, generating reports, and implementing bug fixes for every business unit require time. As adoption rates for your data program grow, there aren’t enough resources to go around.
Even as you hire data analysts with a mix of technical skills and business acumen, a centralized structure begins to break down. When analysts switch from working on a request from marketing one day to finance the next, they inevitably need time to get up to speed. No matter how skilled your data team, your organization encounters switching costs as each analyst works to understand the context of new requests.
As delays mount, your teams may lose confidence in your new data program and look for answers elsewhere. Plus, your hard-to-find generalist analysts may feel the pressure and impact employee retention. To break the bottleneck, you need to extend your data team further into your organization.
Semi-embedded teams offer transitional support to growing organizations
When a centralized data team can no longer keep pace with your needs, you don’t need to immediately reinvent your organizational approach. Before breaking apart your centralized structure, you can temporarily gain the advantages of both with a semi-embedded approach.
With this model, your organization still functions with a centralized team, but it’s divided into separate pods. For example, if your organization has a 10-person team, you divide it into five separate teams of two people primarily focused on sales, product, marketing—wherever your organization needs dedicated support. However, they still report to your VP of analytics and remain grouped in a single unit.
A semi-embedded approach adds some much-needed specialization to your analytics efforts while retaining centralized processes. Along with retaining best practices and shared learning, this model also reduces the potential for duplicated effort. But it may not always be efficient. Since your centralized team no longer shares resources, not every topic-specific pod will be equally busy.
How the semi-embedded approach begins to break down
Though a semi-embedded model has advantages, it ultimately isn’t sustainable. As your analytics team grows more dedicated to their specific topics, it loses the wider context for your business priorities. Plus, as your organization grows, your analytics efforts require more specialized attention.
A 10-person analytics team can be effective if it’s divided between five areas of focus. But at larger organization, which can have as many as a hundred stakeholder teams, a semi-embedded approach breaks down.
A fully embedded approach to analytics requires care to implement
As your organization scales toward data maturity, every functional area of your business requires specialized analytics resources. Teams are using data to inform their decision-making, and they require a dedicated resource to meet their needs.
At a company like Facebook, every product team includes its own analyst, data engineer, and various product managers to address its analytics needs. Or, at smaller firms, teams may include an embedded analyst. They sit with the marketing team, focus solely on their analytics needs, and understand everything tied to their operation from a business perspective.
Though a decentralized or matrixed approach eliminates bottlenecks, it introduces problems from a best practices standpoint. Organizations need to maintain clear lines of communication to prevent duplicated efforts from team to team. Your finance team’s analyst may find an issue with your data that your marketing team is also working to resolve.
To allow your data program to flourish, your organization should establish a center of excellence that includes stakeholders from each team. Made up of employees invested in your data but not necessarily data specialists, this team allows you to retain a centralized resource for data governance and change management.
Managing your data’s support system is as critical as your data
Like every component of your data stack, the organizational structure around your data requires consistent care. As your organization outgrows a centralized model, you also need to divide your data engineering teams from your analysts and data scientists.
As reporting structures change, your lines of communication should follow. Organizations should develop handoff points between disciplines to ensure a clear line of responsibility. Though data maturity often requires a move away from centralized resources, your data analytics needs remain a shared priority.
Services provided
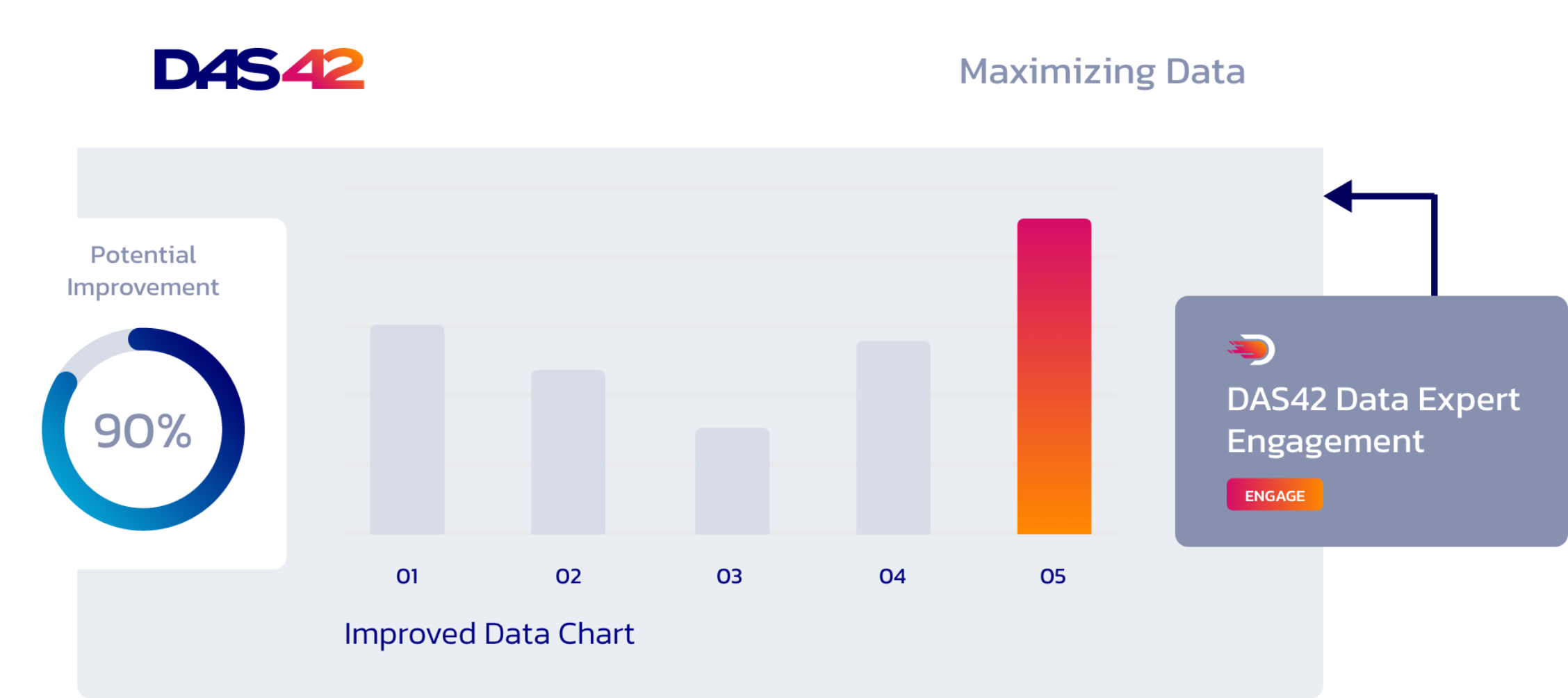
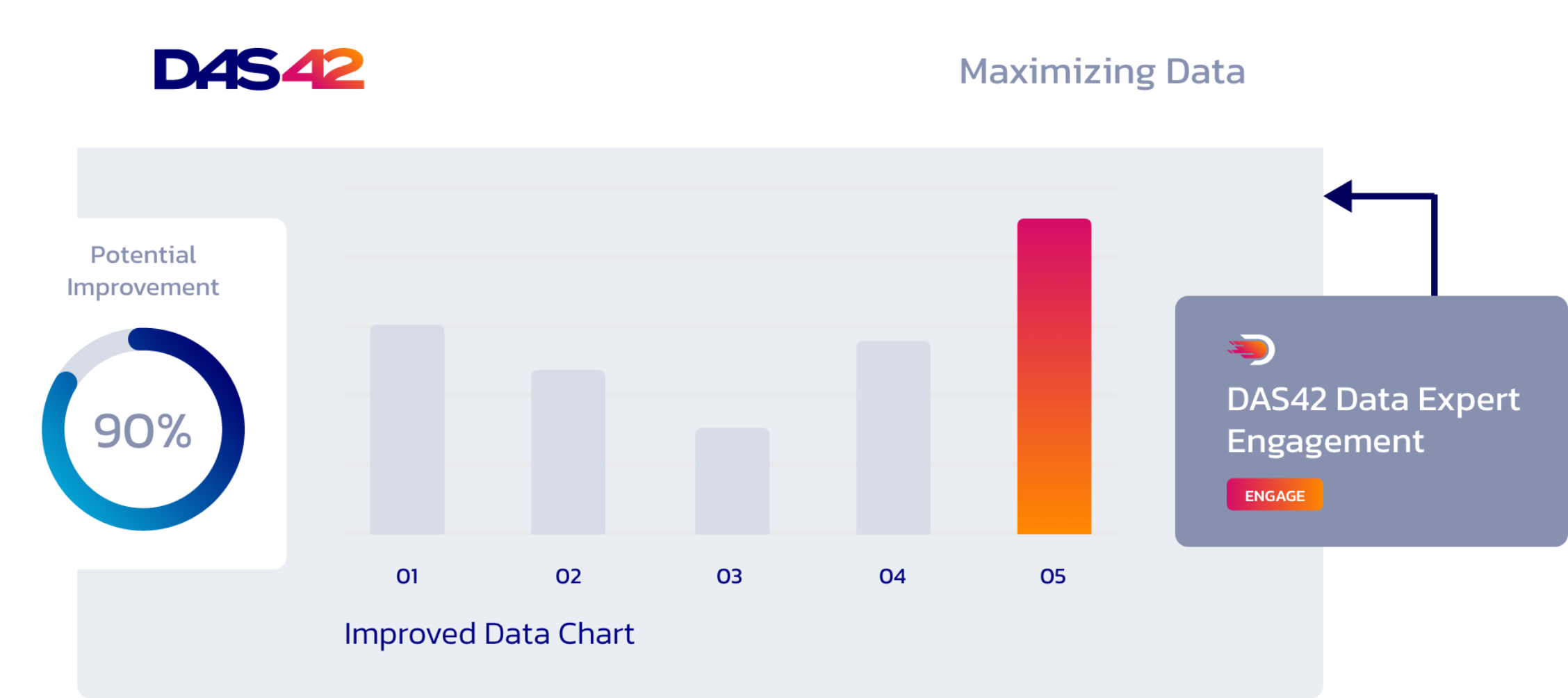