By Teresa Kovich and Barr Moses of Monte Carlo
Everywhere we go in the cloud data space today, we’re hearing one message loud and clear: “you should be thinking about data governance”. It’s a sentiment that we wholeheartedly endorse, but we like to take it a little bit further – you should be thinking about data governance differently.
At DAS42, we offer services that help clients from retail startups to multinational media giants get value from their data quickly and scalably. Monte Carlo provides end-to-end data observability to help data teams discover and resolve their data issues faster—from ML-powered quality monitors and automatic field lineage to on-demand reliability insights and ultra-fast deployments. Together, we help data teams build and scale more reliable systems and data governance strategies.
In this article, we’ll share six of the top reasons that we have seen data governance initiatives fail for even some of the best data teams – and how you can avoid falling into the same traps.
You think data governance is about right and wrong
At a major ridesharing company, two teams had been struggling for months to reconcile their reporting. No matter what they did, they were never able to achieve the same results, even when they insisted that they were defining their metrics in the same way, having queried from the same tables and walked through the code line by line. DAS42 accepted the challenge, and what we found became one of our favorite examples of the criticality of data governance.
One team was using a filter on “mega-region = ‘US and Canada’”. The other team was using a filter on “country-code = 1 (US) OR 32 (Canada)”. These, we were told, were the same thing. But sure enough, we looked at the mapping table and found that country-code 1 included Puerto Rico, but the mega-region ‘US and Canada’ did not.
It wasn’t that one team was right and the other was wrong. It was that neither of them completely understood what was being included in or excluded from their metric.
This is one of the most common data governance errors we see, and it’s led to some of the most pernicious and disheartening data woes for our clients.
It is not enough to point at a query or output and say “this is the source of truth” – to say “this number is right, and any other number is wrong”. This is because fundamentally, data governance is not about right and wrong. Both of the teams mentioned above had numbers that would be valid, depending on the use case – depending on what the stakeholder meant when they said “we want these numbers for US and Canada”.
That’s not to say there’s no such thing as a “wrong” number. Of course, we’ve also seen analysts try to exclude test accounts with a clause like “last name does not contain ‘test’”, which is a terrible shame for real human beings named with surnames like Battesten or Contestanza. (We have yet to encounter a real person named Testy McTesterson, but stranger things have happened.)
But it is not useful to enshrine a number – a metric definition, for example – as right if you are not able to explain what differentiates it from the others.
Shift your mindset from “we need to know which of these numbers are right and wrong” to “we need to understand these numbers, what goes into them, what makes them different, and in what contexts it would be appropriate to use them”.
You over-emphasize executive buy-in
Don’t get us wrong – of course having respected senior team members as champions for your data governance initiatives is a powerful tool in your toolbox. But it’s also absolutely essential to have buy-in from the people handling the data on a day-to-day basis as well—your front-line staff, your engineers, your project managers—the people who will have to make changes to their processes in order for you to fully implement a governed framework.
Before you start trying to secure leadership and stakeholder buy-in, it’s important to be transparent about the current state of your data governance strategy. Consider how you might answer the following questions:
- How do you measure the data quality of the assets your company collects and stores?
- What are the KPIs or overall goals you’re going to hold your data governance strategy accountable for meeting?
- Do you have cross-functional involvement from leadership and data users in other parts of the company?
- Who at the company will be held accountable for meeting your strategy’s KPIs and goals?
- What checks and balances do you have to ensure KPIs are measured correctly and goals can be met?
In the same way that having visibility into your data pipelines makes it easy to ensure high data quality, transparency into both your data governance strategy and its incremental progress will be critical when it comes to keeping everyone informed and accountable on your leadership team.
You think of implementing data governance as a project
There are two primary mistakes data leaders make when it comes to implementing a data governance framework. The first is the “set it and forget it” mentality. They think of data governance as an initiative to be completed, and that once it’s done, they’ll be on to the next. The second mistake is similar, which is the inclination to over-govern. While understandable, both approaches miss the heart of what data governance is intended to be.
Just like data ingestion or quality assurance, data governance is a process. It may require more intentional effort on the front end, but data governance isn’t a project that’s ever truly completed. As your company grows and evolves, your metric definitions will evolve along with it. The problem with the approaches mentioned above is that they don’t leave room for change.
Remember that data governance is less about the right and wrong of your metrics and more about changing your company’s cultural approach to those metrics. Don’t think of data governance as something to be completed—think of data governance as something to be adopted.
You think a tool will do all the heavy lifting
The data landscape is crowded with tools, managed services, methodologies, and frameworks, and we don’t deny that many of them really can help you take your data to the next level. Data observability is one of those. But, as highlighted above, data governance isn’t a single tool, or a workflow you set up once and all your problems are solved—it’s an ongoing process that involves judgment, decision-making, and differentiation.
While data catalogs and other governance solutions often market themselves as the answer to all of your company’s data problems, many data leaders find these tools lacking in even the most rudimentary aspects when it comes to manual requirements.
There are tools and companies that can make things simpler, automate processes, and help you to step outside of your assumptions. But for all the innovation we’ve seen across the data landscape over the last decade, there’s still no technological replacement for the difficult (and immensely gratifying) work of talking about your company, your processes, your definitions, your measurements, and your goals.
You think you can focus on data governance alone
Some of the most earnest stakeholders we’ve seen profess their commitment to implementing data governance by putting it at the top of their priority list – and putting everything else on hold. They want to govern everything, and they want to do it now. This can cause several problems.
First, it can lead to frustration and disenchantment from stakeholders as they fail to see material gains in the form of new deliverables. (Of course, some of us think that data governance is tremendously exciting, but probably not everyone at your company is this particular variety of nerd.)
Second, if you fail to take an iterative approach, practical lessons might come too late. We’ve seen companies “govern everything” and then, a couple weeks after they’ve finished and everything is implemented, see something major that they missed… and they missed it across the board. We mentioned above the criticality of getting buy-in from stakeholders at all levels.
As you start on a data governance journey, choose a few key examples. These might be fundamental to your business – you may need to define your categories or product hierarchies, to determine your net operational revenue or availability rate, or you might need to define what you mean when you use the word “customer”. (How do you exclude those test accounts, anyway?) Choose two or three high-impact areas to govern. Build your governance muscles, and make it sustainable – something that you can do alongside your day-to-day work and “business-as-usual” deliverables.
And give yourself time to learn lessons as you move on to the next, and the next.
You don’t know what data actually matters
Once you’ve identified the first few domains you’d like to focus your energies on, the next step is to ensure that the data you’re governing is actually worth being governed. Not all data is created equal, and in today’s economic climate, it is not inaccurate to say that some data is worth more than others. For instance, data forecasting your company’s revenue next quarter is probably more worthy of your attention than a duplicate table sitting in a dusty corner of your Snowflake warehouse.
Before you roll out your governance strategy, identify what data actually matters most to your business and prioritize accordingly. Having visibility into your most critical assets – no matter what stage of the pipeline they’re in – can ensure that your team is a) spending time building a data governance program for data the business is actually using and b) tell you if the data is available, fresh, and, most importantly, accurate.
One way to do this? Set service-level agreements (SLAs) and service-level indicators (SLIs) for data assets with the most eyeballs, for instance, the table feeding your CFO’s quarterly metrics dashboard or that Salesforce data informing your ad campaigns.
Setting data reliability SLAs helps build trust and strengthen relationships between your data, your data team, and downstream consumers – whether that’s your customers or cross-functional teams at your company. Without these clearly defined metrics, consumers may make flawed assumptions or rely on anecdotal evidence about the reliability and trustworthiness of your data platform. In other words, data SLAs help your organization be more “data-driven” about data – and in turn, data governance.
So, let’s get started.
For most organizations, data governance is relegated to a few lone wolves responsible for convincing an entire team of “numbers people” to care about something that is inherently difficult to quantify. If it sounds like a trap, it’s because it is one – but it doesn’t have to be.
At the end of the day, the goal of your data governance strategy will be to ensure that teams across the entire company feel empowered to use data, and the only way to empower is to build trust and educate.
Our biggest suggestion: start your governance initiative on a few key functional areas (land and expand), tracking for a few key SLAs, and across a handful of critical data assets. In a world where bigger (data) is always better, sometimes it pays to start small.
Interested in learning more? Reach out to DAS42 or Monte Carlo to embark on your data governance journey.
Services provided
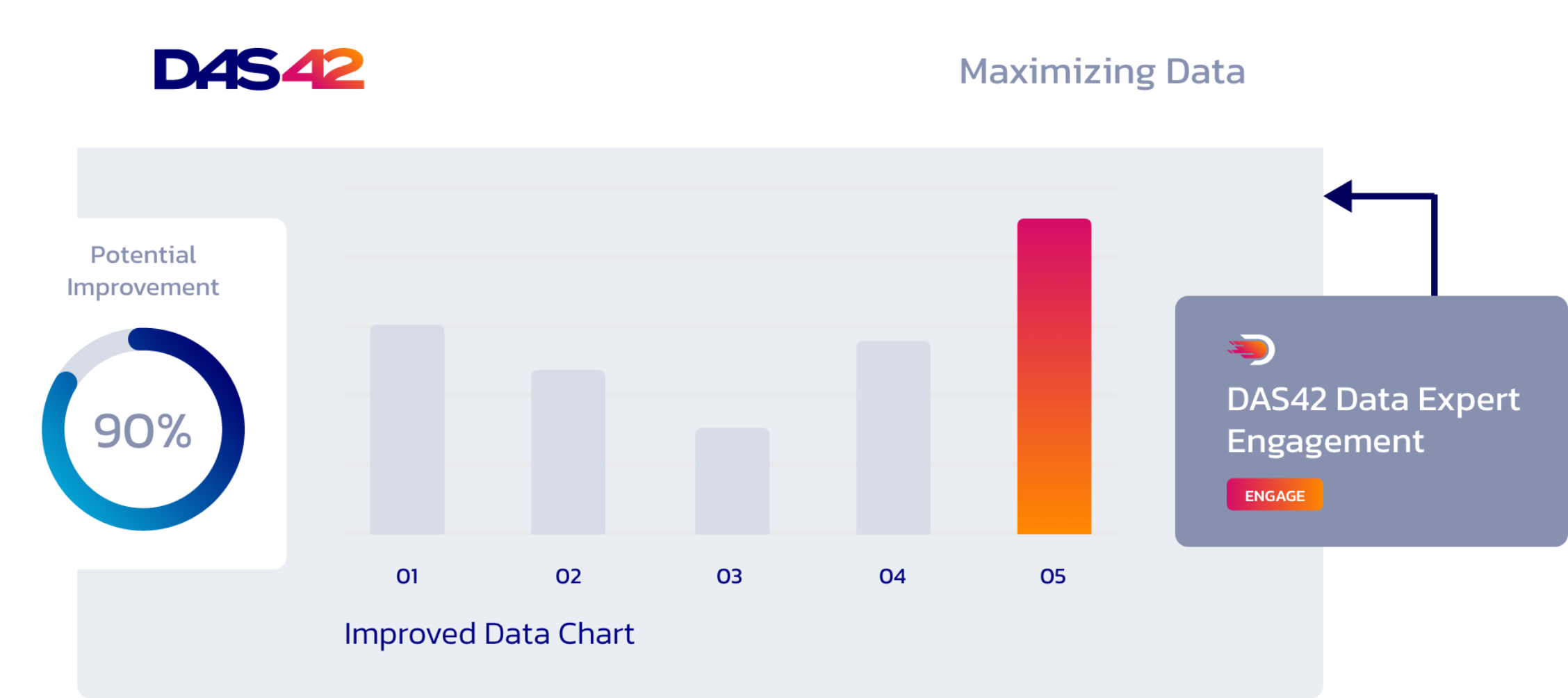
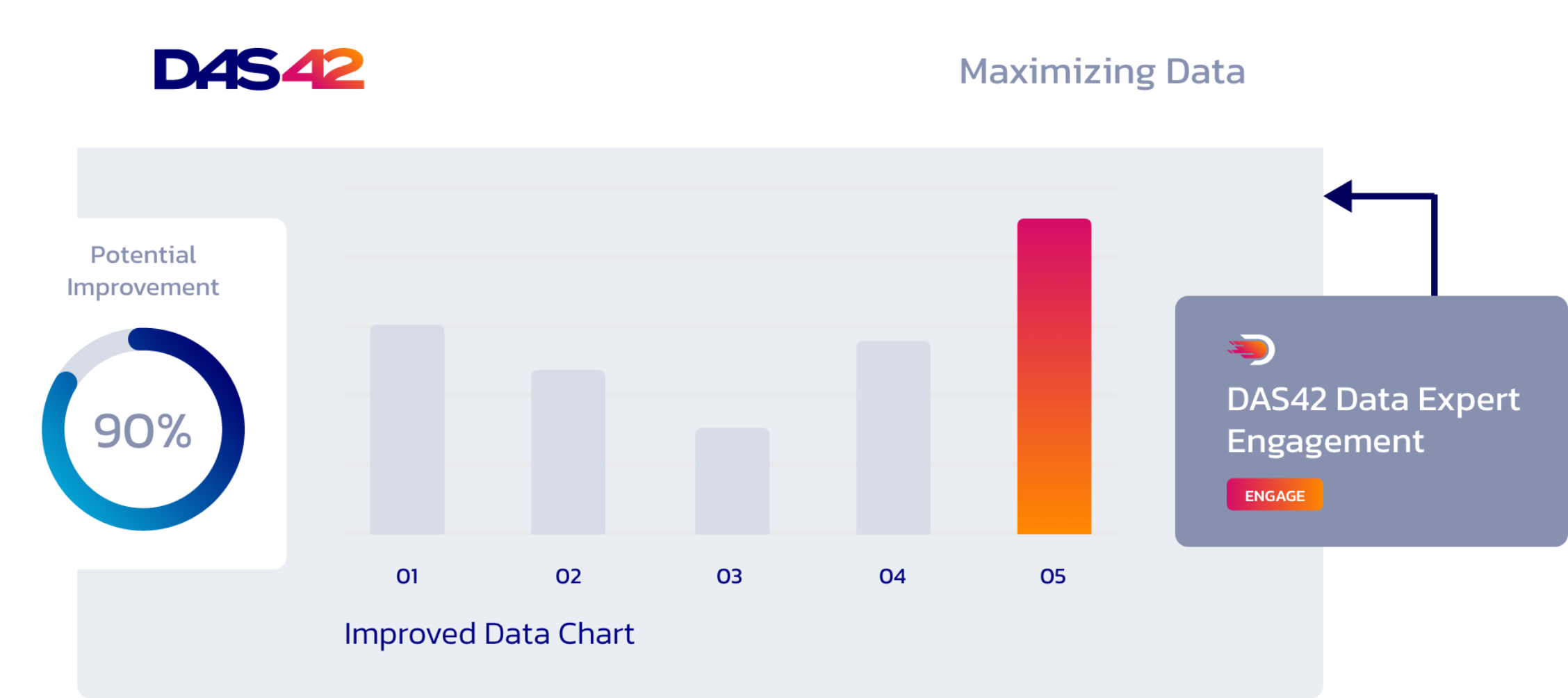