Strategic Insights and Analytics Solutions for Reducing Churn in the Telecommunications Industry
Telecommunications companies are constantly competing, striving to retain their customer base in the face of growing challenges. One of the key strategies in maintaining a solid customer base is predicting and preventing customer churn. Predictive analytics provide a powerful tool for telecommunications companies to identify at-risk customers and proactively implement retention strategies.
Understanding Customer Churn
Customer churn, or the rate at which customers stop using a company’s services, is a critical metric in the telecommunications industry. High churn rates reflect customer dissatisfaction and result in significant revenue losses. Understanding the reasons behind churn and identifying at-risk customers before they leave can give a company a competitive advantage over another.
- Involuntary Churn:
Involuntary or “passive” churn occurs when a customer’s contract expires without renewal. Sometimes, it’s due to the customer’s forgetfulness rather than a deliberate choice to leave. Other times, this churn happens due to circumstances beyond the customer’s control, such as financial issues leading to service cancellation or relocation to areas outside the service coverage. - Voluntary Churn:
Contrary to passive churn, voluntary or “active” churn is a deliberate decision by the customer to end their relationship with the company. It often results from poor service quality, customer service issues, or more appealing competitor offers. - Non-Payment Churn:
Off-cycle discontinuation of a service due to missed or late payments is non-payment churn. It could be intentional, due to card expiration, or due to a customer’s financial constraints.
Understanding these churn types helps telecom companies tailor their predictive analytics strategies more effectively.
The Role of Predictive Analytics
Predictive analytics utilizes governed data, statistical algorithms, and machine learning techniques to identify the likelihood of future outcomes based on historical data. In the context of churn prediction, these analytics help understand customer behavior, preferences, and dissatisfaction signs. Typically, the implementation of predictive analytics follows this sequence:
> Data Collection and Integration
Telecom companies collect vast amounts of data from various sources, such as service usage, call records or other customer service interactions, billing information, and social media engagement. Data collection and integration are crucial to combine all this information into a unified dataset. This step will involve data cleansing and transformation to ensure data quality and consistency.
> Data Storage and Analysis
Once the raw data is collected, it must have a secure and accessible location for analysis. Setting up databases or data warehouses that can efficiently handle large volumes of data is the next necessary step. Cloud-based databases, such as Snowflake, best fit this purpose for a modern data storage solution. The focus is on building a robust infrastructure that supports dynamic data processing and analysis, allowing telecom companies to quickly identify trends and patterns related to customer churn.
> Prediction and Testing
Once data is conformed and governed, machine learning algorithms that use historical and current data are applied to systematically identify combinations of trends at a level of complexity and thoroughness not typically attainable by standard human analysis. One critical step is to collect and account for data that documents past churn–reduction campaigns and their success or failure so that the effectiveness of previous strategies can be assessed.
> Visualization and Reporting
This step involves converting the analyzed data into visual reports and dashboards. These visualizations help in making the data understandable and actionable. They provide a clear picture of customer behaviors and churn patterns, making it easier for decision-makers to identify at-risk customer segments, understand the factors driving churn, and tailor the recommendations to each segment.
> Decision-Making and Action
The final step is using the insights gained from predictive analytics to make informed decisions and take proactive actions to reduce churn. Some examples of strategies for customer retention include customer personalization, targeted marketing campaigns, and improvements in service delivery. Translating data-driven insights into practical, effective actions that enhance customer satisfaction and loyalty is key.
Challenges and Considerations
While predictive analytics presents immense potential in transforming customer retention strategies in telecommunications, it has challenges and considerations. These include:
- Data Privacy and Security:
With the collection of vast amounts of customer data, maintaining privacy and ensuring security is paramount. Telecom companies must adhere to strict data protection regulations set forth by the Federal Communications Commission (FCC). The challenge lies in responsibly managing sensitive customer information while extracting valuable insights. Strategic anonymization or de–identification can be applied in some cases, and in others, especially sensitive data elements, such as credit card numbers that are not meaningful for analysis, may be scrubbed from the data altogether. - Data Accuracy and Relevance:
The effectiveness of predictive analytics heavily relies on the quality of the data used. Inaccurate or outdated data can lead to erroneous predictions and misguided strategies. Companies must ensure their data is accurate, up-to-date, and relevant to the specific churn factors they aim to analyze. Joining data from multiple sources or enriching data with third-party datasets also requires applying identity resolution techniques. - Skill Set and Resource Allocation:
Implementing predictive analytics requires a specific skill set and resources, including data scientists, analysts, and the right technologies. Finding and retaining such talent can be challenging, as is allocating the resources required for continuous data analysis and strategy implementation.
Partnering with DAS42
Partnering with a knowledgeable and experienced data consulting firm like DAS42 can be invaluable in implementing predictive analytics for churn prevention. DAS42’s comprehensive solutions address the key challenges in predictive analytics, ensuring telecom companies can harness the power of their data effectively and securely. By collaborating with DAS42, companies gain a data analytics consultancy and a strategic partner committed to their success in the dynamic telecommunications market.
DAS42 is a premier data and analytics consultancy with a modern point of view. We specialize in solving some of the most complex business challenges for the world’s most successful companies. As a Snowflake Elite Partner, DAS42 crafts customized strategies that create a single source of truth and enable enhanced and faster decision-making. DAS42 has a presence across the U.S. with primary offices in New York City and Denver. Connect with us at das42.com and stay updated on LinkedIn. Join us today on our journey to help you realize the possibilities of transforming your business through data and analytics.
Services provided
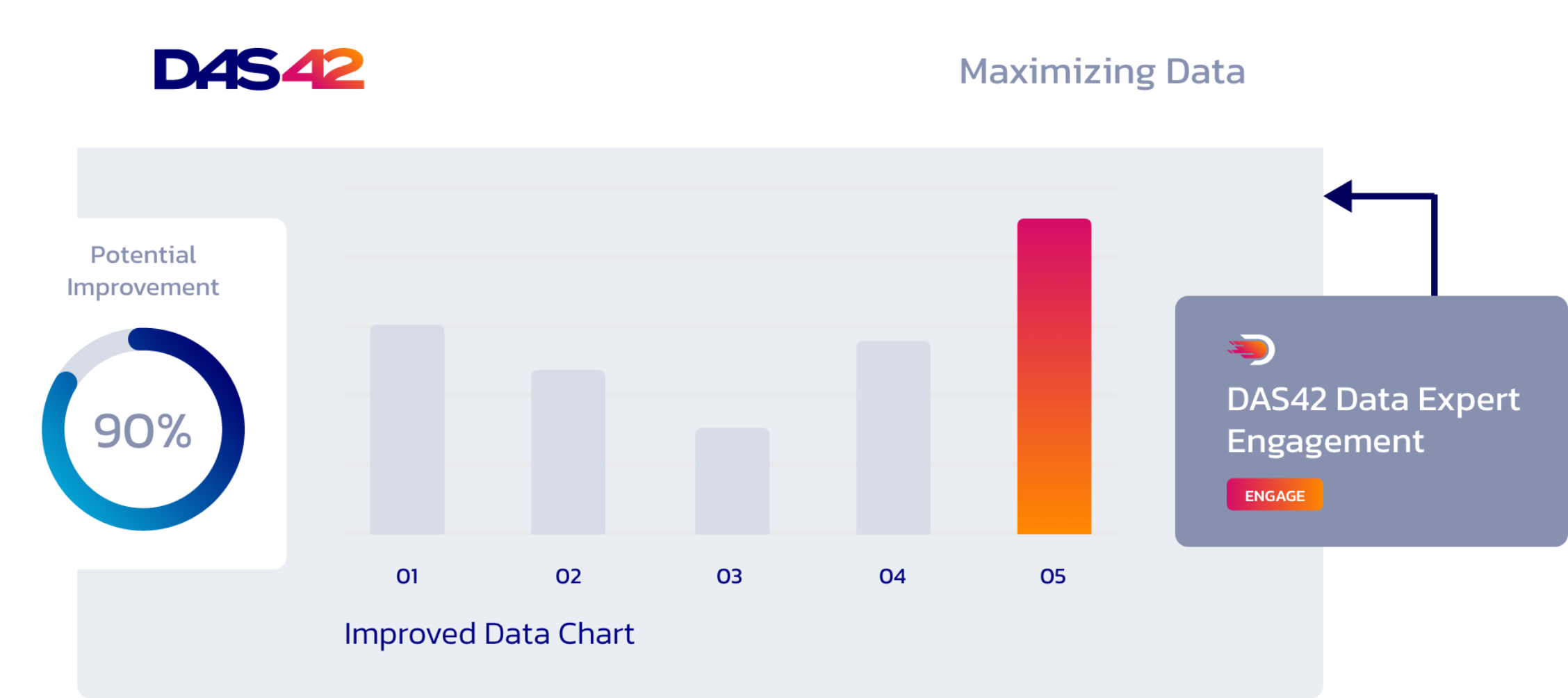
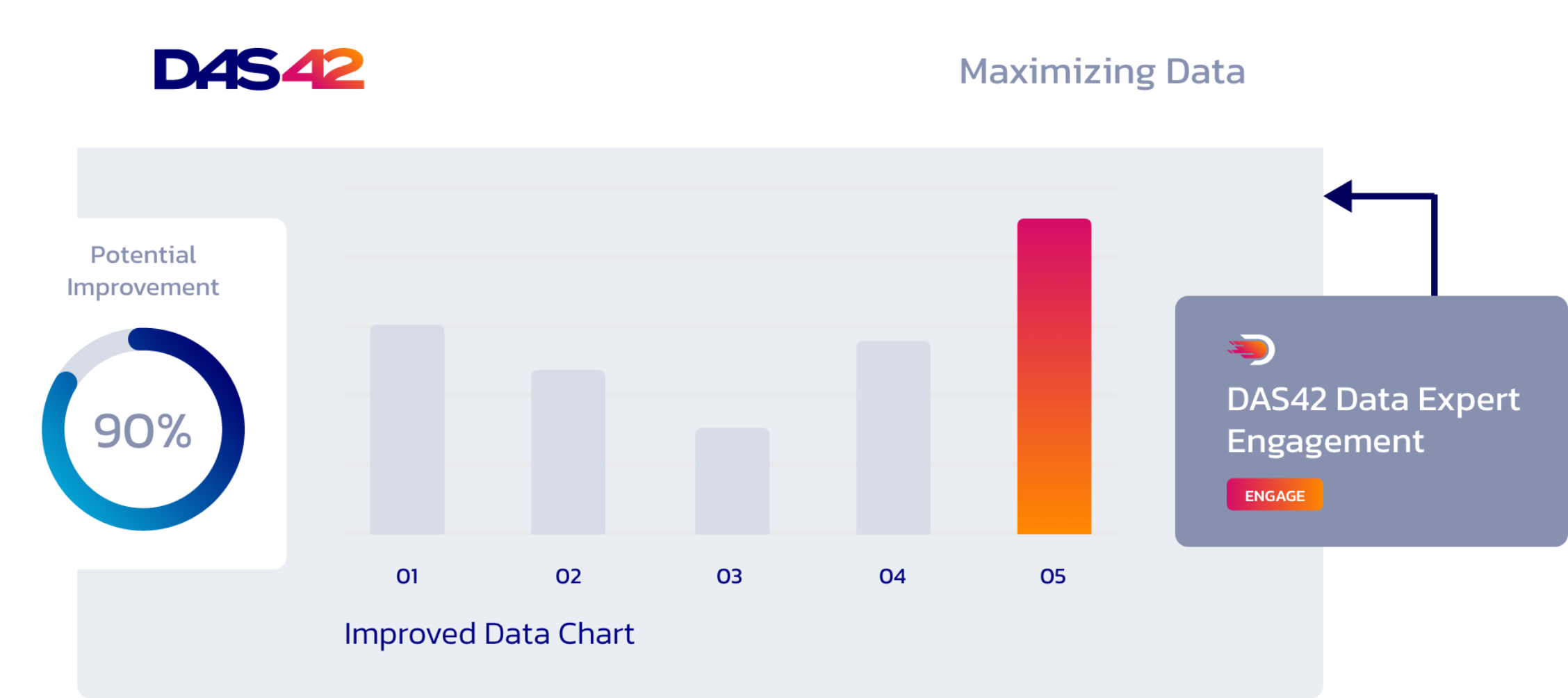