If you watch enough commercials, you quickly get the idea that machine learning has already begun transforming our lives. And, by extension, your business can’t risk falling behind.
Not so fast. Whenever a buzzed-about new technology arrives, companies often feel like they’re missing a transformative addition to their existing processes. But despite all the FOMO around machine learning, it’s not a panacea that will take your business to new heights. For the most part, its use cases remain limited and very specific.
Right now, machine learning is too costly and immature a technology to experiment with and determine its feasibility for your business. However, there are promising signs that point to a future where machine learning will live up to its considerable hype.
In all likelihood, machine learning isn’t right for your business – yet. But before you can know whether the technology could satisfy your needs, you need a solid foundation in place. As with so many other business initiatives, that first step starts with your data.
How machine learning is applied today
From eCommerce to finance, companies in an array of industries are using machine learning to save time and generate insights in a new way.
The idea of machine learning and artificial intelligence feels grounded in science fiction. But its practical use remains grounded in reality. The industry has yet to coalesce around a proven set of tools or methodology, and the business applications are so far very narrow.
At its root, machine learning is built around using programming to analyze a large set of data and draw conclusions based on specific patterns. Still primarily in its infancy, the technology is most often used in three ways:
1. Categorization
Machine learning is used to program an algorithm that assigns a label to information that meets specific criteria. For example, banking transactions that fail to meet certain conditions are flagged as fraudulent, and those that do satisfy the criteria are not.
2. Image analysis
Complex algorithms allow machine learning to determine whether images or videos meet defined specifications. By being programmed to identify visible signs of wear and potential failure in equipment such as power lines, machine learning allows companies to perform predictive maintenance and prevent costly outages.
3. Sentiment analysis
Also qualifying as a categorization problem, sentiment analysis applies an algorithm that analyzes text for positive or negative associations. By programming machine learning to recognize emotions in language, it can provide responses such as product recommendations without human input.
When applied to user behavior, machine learning can recognize if a person enjoyed something like “Game of Thrones” and, in the case of a streaming or retail provider, recommend similar products.
Machine learning applications will simplify in time
Given machine learning is still in the earliest stages of its adoption curve, we’re not yet seeing groundbreaking applications in the industry. Though the technology’s current facility with pattern recognition and prediction provides a valuable service, it will become far more accessible with time.
Rather than reinventing the wheel by designing an algorithm to suit your business, you’ll soon be able to buy an off-the-shelf software solution. If you’re a financial services company, you’ll be able to analyze transactions and spot fraud. Or, in the case of eCommerce, you can feed a tool your sales data and generate product recommendations.
But to produce machine learning applications like these now, companies often underestimate the expertise they require. For example, a residential building management firm wants to streamline how apartment residents are notified a package has arrived. Using machine learning, the company wanted the ability to scan a package, identify its owner, and send residents a text indicating its arrival.
The idea is a cool use case, and suits the common application of image analysis and processing. But even with a data scientist on staff, they may be able to develop a data model and not know how to deploy it. Unfortunately, machine learning models require software engineering skills, DevOps skills, and testing to effectively implement.
Combining these skills is an expensive undertaking for what isn’t a core part of the building management firm’s business. Once it’s launched, maybe the application leads to new revenue, maybe not. But given the required investment, companies are better off waiting until off-the-shelf tools arrive in a matter of years.
MLOps points to a promising future for machine learning
While the software and tooling required for machine learning remains a work in progress, it still has potential for a bright future. One of the clearest indicators of that future coming into focus is the rise of a new discipline called machine learning operations, or MLOps.
Typically overlooked as companies envision machine learning use cases, MLOps is one of the most exciting developments in the industry. MLOps is less focused on data science and more on the DevOps and software engineering skills that machine learning requires as well as knowledge of statistics and computer science. At the forefront of developing machine learning models, MLOps marks the start of an effort to focus on the processes the technology needs to be effective.
With the technology in its early stages, only early adopters like Amazon have the resources to focus on MLOps. Because the discipline is still young, MLOps specialists are in high demand, and they’re often quickly snapped up by these larger firms. Even if your organization has an idea for a machine learning application, you’ll have difficulty finding the skills and bandwidth to deploy it.
However, given that MLOps is a new discipline, multiple young companies are coming online to coalesce around establishing the right tools and best practices. As MLOps grows, so will the possibilities for companies to experiment with machine learning more affordably.
Data storage platforms are evolving toward machine learning
As machine learning continues to evolve, there aren’t many effective ways to integrate machine learning into your investment in a cloud-based data warehouse. But your options are growing.
Google’s BigQuery has released BigQuery ML, which allows you to create machine learning models without the need of other tools. And Snowflake now allows integration with products like H2O.ai, which promises to similarly enable use of machine learning.
But these are still early developments that will also require specialized skills. H2O.ai requires Java and Python coding, as well as the understanding of how machine learning works. Plus, it requires MLOps knowledge to deploy it into production.
However, as the technology grows, more integrations like these that lower the barrier of entry to machine learning will follow. In five to ten years, the technology could become more fully integrated and close to plug-and-play.
Machine learning requires a modern data program
With expansive potential, machine learning is frequently presented as cutting edge technology that will reshape the future of your business. While that may be proven true, its time hasn’t yet come. From the standpoint of creating applications that are cost-effective and break new ground, machine learning hasn’t reached enterprise-level adoption.
For most companies, you’re better off focusing on the basics. Before machine learning enters the conversation, you must ensure you have access to a consistent and accurate set of data. Once you’ve implemented a modern data stack, you’re probably closer to the beginning of your data analytics journey than you realize.
For now, it’s really just the early adopters with the right skills and resources that can experiment with this technology. For everyone else, given the prospect of better integrations and off-the-shelf tools ahead, you’re better off waiting for the future a little longer.
Services provided
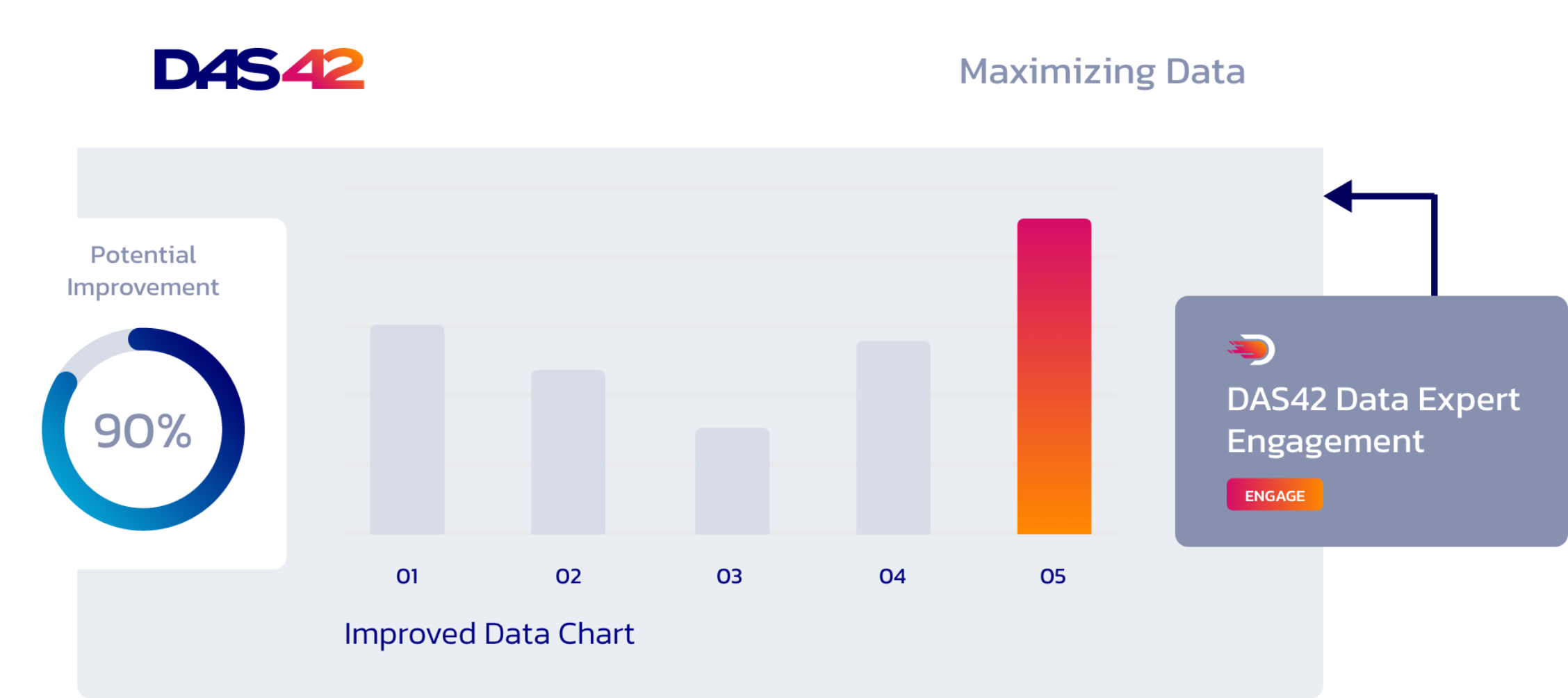
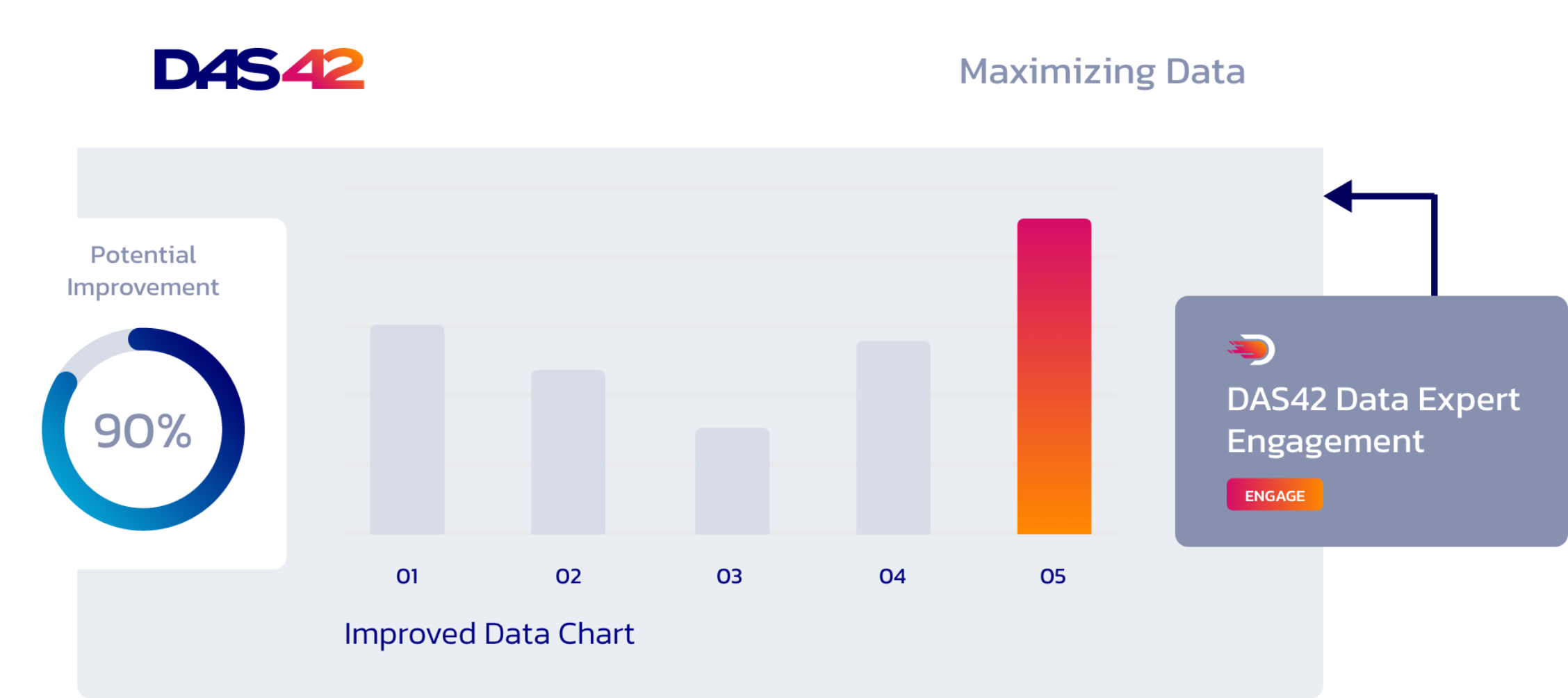